Utilizing AI for Effective Risk Assessment
- Feb 16
- 3 min read
Updated: Feb 19
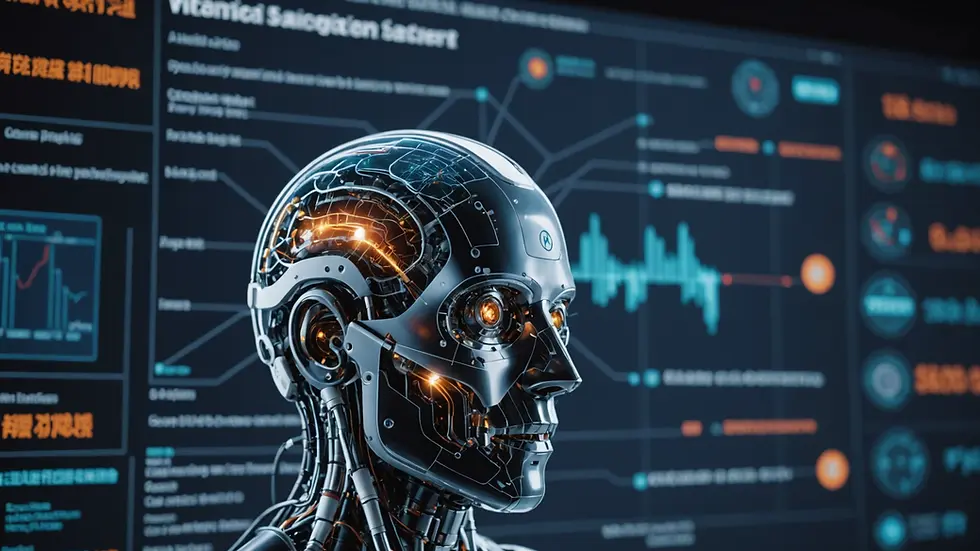
Risk assessment is a critical component of health, safety, and environmental (HSE) management in the oil and gas industry. Given the high-risk nature of operations, including offshore drilling, refinery processes, and pipeline transportation, ensuring accurate and efficient risk assessments is paramount.
Traditional risk assessments rely on historical data, human judgment, and standardized methodologies, but these approaches can be time-consuming and prone to human error. Artificial Intelligence (AI) is revolutionizing risk assessment by providing data-driven insights, predictive analytics, and automation to enhance safety and efficiency.
The Role of AI in Risk Assessment
AI technologies, including machine learning (ML), natural language processing (NLP), and computer vision, are transforming how risks are identified, analyzed, and mitigated in the oil and gas sector. These technologies enable:
Predictive Analytics – AI can analyze historical incident reports, sensor data, and operational parameters to predict potential hazards before they occur.
Automated Risk Identification – AI-powered systems can process vast amounts of real-time data from IoT-enabled equipment, detecting early warning signs of equipment failure or hazardous conditions.
Enhanced Decision-Making – AI algorithms can provide risk scores and recommendations, assisting HSE professionals in making informed decisions to mitigate risks effectively.
How AI Improves Efficiency in Risk Assessments
Real-Time Data Processing: AI enables the continuous monitoring of operations through real-time data streams from sensors, drones, and surveillance cameras. For example, AI-powered drones can inspect offshore platforms, pipelines, and storage tanks, identifying potential safety risks such as corrosion, leaks, or structural weaknesses without human intervention.
Reduced Human Error: AI minimizes the subjectivity and variability associated with human-led risk assessments. By standardizing risk evaluation processes, AI ensures consistent and objective risk assessment outcomes.
Faster Risk Analysis: AI-driven models can process and analyze large datasets in minutes, reducing the time required to conduct comprehensive risk assessments. This enables companies to take proactive measures and avoid costly accidents and downtime.
Improved Regulatory Compliance: AI tools can help organizations stay compliant with evolving industry regulations by continuously monitoring compliance requirements and flagging potential non-conformities.
AI Applications in Oil and Gas Risk Assessments
AI in Equipment Failure Prediction
Machine learning models analyze sensor data from drilling rigs, refineries, and pipelines to detect anomalies that may indicate equipment failure. For instance, AI can predict pump failures or pressure anomalies in pipelines, allowing for predictive maintenance and reducing unplanned shutdowns.
Hazard Identification Through Computer Vision
AI-driven computer vision systems can analyze video feeds to identify hazardous conditions such as gas leaks, fire hazards, or improper use of personal protective equipment (PPE). These systems improve workplace safety by alerting operators and safety managers in real time.
AI in Incident Analysis
AI can analyze historical incident reports and identify patterns leading to accidents. By leveraging NLP, AI can extract key insights from textual safety reports and suggest preventive measures, reducing the recurrence of similar incidents.
AI in Emergency Response Planning
AI models can simulate emergency scenarios based on historical data and real-time conditions, helping companies develop more effective emergency response plans. For example, AI can predict the impact of an oil spill or explosion and recommend optimal containment strategies.
Challenges and Considerations
While AI presents significant benefits, its implementation in risk assessments comes with challenges:
Data Quality and Integration: AI models require high-quality, structured data from multiple sources. Integrating data from legacy systems, sensors, and safety reports can be complex.
Cybersecurity Risks: AI-powered systems rely on interconnected networks, making them susceptible to cyber threats. To prevent data breaches, robust cybersecurity measures must be in place.
Human Oversight: AI should complement, not replace, human expertise. HSE professionals must validate AI-generated insights and ensure that automated recommendations align with operational realities.
The oil and gas industry can significantly improve risk assessment efficiency and accuracy through AI-driven technologies. By leveraging predictive analytics, real-time data processing, and automation, AI enhances safety, reduces downtime, and ensures regulatory compliance. However, successful implementation requires addressing challenges such as data integration, cybersecurity, and human oversight. As AI continues to evolve, it will play an increasingly vital role in safeguarding the industry's workforce, assets, and the environment.