Data Quality Matters in GenAI
- Feb 23
- 3 min read
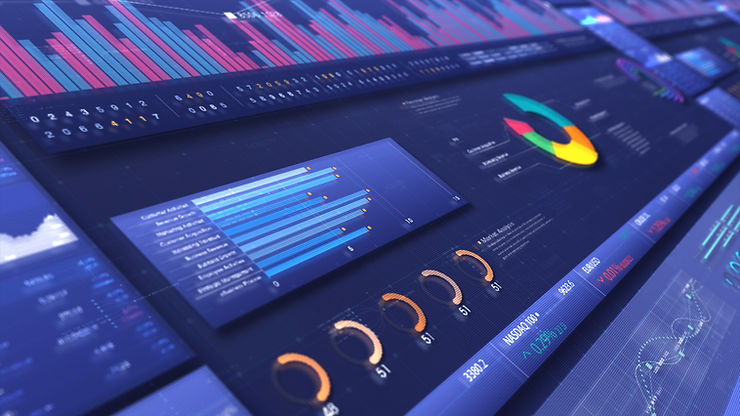
In the realm of artificial intelligence, particularly with large language models (LLMs) and generative AI, the phrase “garbage in, garbage out” has never been more pertinent. As a Project and HSE (Health, Safety, and Environment) Consultant, you appreciate the importance of high-quality data as the foundation for effective decision-making and operational efficiency.
This article explores the intersection of data quality and generative AI, emphasizing how a well-structured data approach can lead to meaningful insights and improved project outcomes.
Understanding Generative AI and Large Language Models
Generative AI, driven by models like ChatGPT and others, enables the creation of text, images, and other media based on learned patterns from vast datasets. These capabilities can be transformative in industries such as construction, energy, and manufacturing, where data-driven decisions are critical.
LLMs assist in drafting reports, automating responses, and even generating safety protocols. However, the effectiveness of these applications is directly influenced by the quality of input data.
The Importance of Quality Data
Precision in Outputs: High-quality data ensures that the outputs generated by AI systems are accurate and relevant. In HSE consulting, this precision can mean the difference between robust safety protocols and ineffective measures that might expose workers to risks.
Contextual Relevance: Large language models thrive on context. If the input data is rich and contextually relevant, the AI can produce outputs that truly resonate with specific organizational needs. For instance, using historical data from past projects can enhance predictive modeling for future risks.
Mitigating Bias: Poor quality data often carries inherent biases, which can perpetuate errors in decision-making. By ensuring a diverse and representative dataset, organizations can promote fairness and reliability in AI-generated insights.
Enhancing Reliability: In industries where safety is paramount, the reliability of AI-generated outputs can aid in compliance and risk management. Robust data enhances model reliability, ultimately leading to safer work environments.
Feedback Loops: Continuous feedback from operating environments can improve AI models over time. Establishing consistent data collection practices ensures that the input is not only current but also reflective of changing conditions and regulations.
Implementing an In-House Solution
As you prepare an in-house solution for leveraging generative AI within your organization, consider the following strategies:
Data Governance Framework: Establish a governance framework to maintain data integrity. This should include guidelines for data collection, processing, and validation to ensure that only high-quality data enters your systems.
Training and Resources: Invest in training staff to understand the importance of data quality. Encouraging a culture that prioritizes accurate and responsible data handling will pay dividends in the long run.
Collaboration Across Departments: Foster collaboration between departments to ensure that the data being fed into AI systems is comprehensive. Input from HSE, project management, and operational teams can provide valuable perspectives that enhance data quality.
Regular Reviews and Updates: Set up a system for regularly reviewing the data used in AI applications. This ensures that the information remains relevant and that any potential issues can be promptly addressed.
Pilot Testing: Start small with pilot projects to test the effectiveness of your AI implementations, gradually scaling up as data quality improves and models become more refined.
In an era increasingly dominated by artificial intelligence, the adage “garbage in, garbage out” serves as a crucial reminder for organizations leveraging generative AI. As you develop your in-house solution, focus on creating a robust data ecosystem that prioritizes quality and relevance. By doing so, you can harness the full potential of large language models and generative AI, driving more effective project outcomes and significantly enhancing HSE initiatives.
With the right approach, generative AI can not only optimize processes but also foster a culture of safety and excellence in your organization. As you embark on this journey, remember that the value of AI is only as good as the data that feeds it.